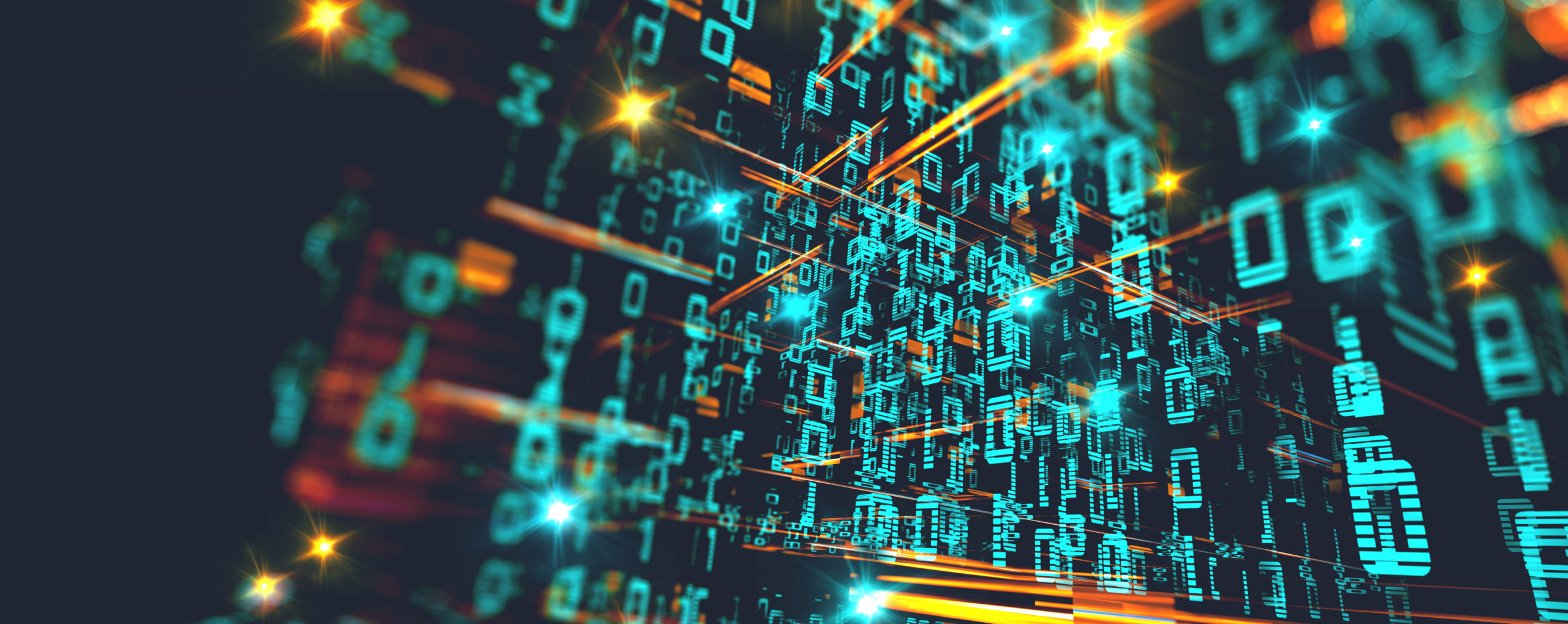
Big Data Doesn’t Always Mean Good Data
Two quintillion bytes (18 zeros) of data is created each day. From a shopping rewards card to laptop internet browsing, mobile gaming to someone’s route to and from work, data analysts have more information than ever before. Effectively analyzing this data is becoming a primary component of managing a business in the 21st century and can improve the practices of organizations large and small. Forbes says that 53% of companies were adopting big data analytics in 2017, and that number can only grow. The question is when does big data become uncontrollable, unusable, and uninterpretable? When is Big Data not necessarily Good Data?
Let’s take an example. You are interested in running for city council in your hometown. You correctly assert that the first task should be to learn about the electorate. What data should you start with? You don’t want to overload your computer (it has very little storage space), so you vow not to collect too much. You also need to be out canvasing, so time spent weeding through messy data is wasted time. You resolve to only download a voting list of the people within your ward who have voted in the past two elections and also gather some information on past ballot issue to gauge what is important. Maybe you keep a short survey as you canvas. The key is to only collect exactly what you need, over the exact time period and geographic location that is relevant! Anything more is wasted time and space.
The above example may seem obvious. However, many businesses struggle with thinking about exactly what data they should be collecting and using. They know in an era of big data it should be leveraged, but to what extent? The candidate’s small storage space is a good representation of most small- to medium-sized companies. The candidate’s recognition to only capture certain data is a reflection of the conversations organizations should be having around data relevancy and how that data is used to illustrate performance (sales, advertising, etc). You don’t need to collect data from 30 years ago if you plan on analyzing what current trends are popular, just like there is no need to collect average gas mileage if you are interested in the most popular car colors. It is little decisions like this that make or break the bank when it comes to big data usage and analytical success. And remember – if you’re not quantifying your efforts, how do you know they’re working?